AI in Women's Imaging: Hidden Truth and Big Reveals
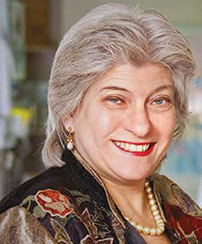
|
Marcia C. Javitt
Chair of Radiology, Rambam Healthcare Campus; Section Editor for Women's Imaging, AJR |
Artificial intelligence (AI) algorithms are under development to interpret datasets to perform tasks for which a computer receives rules or patterns for search. Machine learning is a part of AI in which computer algorithms and statistical models perform a specific task using patterns and training data such that performance improves as more and more actual data are presented. Deep learning is a subset of machine learning in which multilayered processing is performed, such as with convolutional neural networks, to directly interpret unstructured or unlabeled data without supervision.
Breast imaging is likely to be the most successful imaging subspecialty to adopt the principles and practice of augmented intelligence or AI. The infrastructure is already in place. The Mammography Quality Standards Act (MQSA), which outlines performance metrics, was mandated in 1994 and has been updated since then. Risk stratification for mammography based on pattern recognition is standardized with a structured approach using BI-RADS for breast imaging. Breast imaging is digital and in PACS, which makes for streamlined feature analysis and data extraction. Computer-aided detection has been relatively widely used since its approval by the U.S. Food and Drug Administration in 1998. There are training data sets and tumor registries available for training and validation of proposed AI algorithms.
Because the deluge of data in medical imaging is ever growing, even the most capable imaging specialists are reaching human limits for data extraction. We desperately need help with prolific requirements for data extraction, analysis, and pattern recognition. AI has the potential to become a physician extender by automating some of this work by finding previously hidden but important information. It can enhance and improve our performance, thereby enabling us to better serve our patients.
In addition to assistance with faster image interpretation, AI has the potential to speed up workflow in breast and medical imaging while improving cancer detection at screening. Use of computer-aided diagnosis should reduce the number of missed breast cancers, which has been estimated at 20% and higher from interval and screen-detected cancers in retrospect.
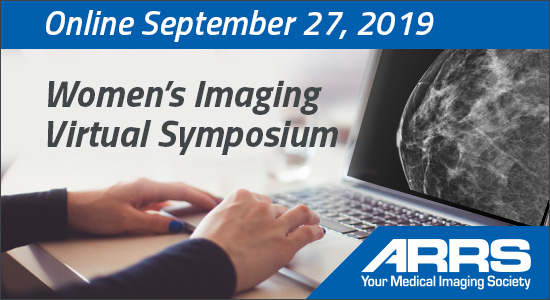
|
One interesting question relevant to breast imaging is whether or not machine learning can add value to breast cancer risk assessment. Machine learning applied to genetic profiles holds promise for generating more accurate risk profiles using information gleaned from single nucleotide polymorphisms. Imaging biomarkers may likewise be incorporated into risk profile. For example, increased breast density, a known risk factor for the development of breast cancer, can be categorized using texture analysis to improve risk stratification. There are already commercial products in use to perform automated mammographic breast density assessment.
When compared with human readers, automated breast density assessment has been found to have less interobserver variability but similar accuracy. Increased breast density is not only a known risk factor for the development of breast cancer but also can mask cancers that are hidden in dense tissue.
As pointed out by participant Toula Destounis in a recent AJR webinar titled “The Value Proposition for Artificial Intelligence in Women's Imaging,” the American College of Radiology started its Data Science Institute to develop algorithms in AI that can assist with lesion detection, characterization, and treatment selection. The Institute will also examine the safety, efficacy, and clinical applicability of such algorithms.
Witness the fact that on March 27, 2019, the U.S. Food and Drug Administration issued a new amendment to the MQSA for breast cancer screening. The changes will require that patients receive a lay letter with information about their breast density and the appurtenant risks. In addition, breast imaging reports to referring providers will also communicate more information about patients’ risks of increased density and breast cancer when appropriate. Improved communication should improve the patient’s and her health care provider’s preparedness to make appropriate management decisions, such as supplemental screening with other modalities for women with increased breast density on screening mammography. In 2019, 37 states have laws requiring that patients receive information about mammographic breast density generally, with fewer requiring that patients receive their own personal breast density information.
Further to this discussion, some of the most exciting research being done is in radiomics of breast cancer. In this era of radiomics, clinical decision-making will be based on harmonizing clinical and imaging biomarkers to achieve personalized patient care. A multiparametric approach is evolving in which lesion detection, tissue characterization, risk stratification using molecular subtypes, treatment choices, prognosis, response to therapy, and prediction of recurrence risk are dependent on integration of clinical and imaging biomarkers. Clinical, histologic, and genomic information about a patient are married to feature extraction, segmentation, and intelligent data management to offer new insights. Precision medicine is becoming a realistic future prospect and will benefit from implementation of AI algorithms. The intelligent use of radiomics should also enable cost minimization and reduced overdiagnosis and overtreatment from screening.
As we move from screening based on the calendar to personalized care, can we achieve risk-based breast cancer screening based on modeling? The published literature is unclear so far, largely because the models are complex, varied, and without standardization. As pointed out by participant Linda Moy in the AJR webinar mentioned earlier, the Breast Cancer Surveillance Consortium case control study found that the addition of breast density to risk models helps to identify women with high risk.
However, another study of more than 15,000 patients suggested that more variables are needed to perform better risk assessment. Although AI-driven, risk-based screening will require further development before it can be implemented without harm, we welcome the opportunity to use such tools to increase accuracy, improve breast cancer detection, and relieve the exhaustion associated with cognitively demanding and ever-increasing workloads in the clinical practice of breast imaging today.